James Zou

James Zou is an assistant professor of Biomedical Data Science, CS and EE at Stanford University. He is also an inaugural Chan-Zuckerberg Investigator and is the faculty director of the Stanford AI for Health program. His group develops state-of-the-art machine learning algorithms motivated by biomedical and health applications. His research have been published in Nature, Cell, PNAS, Nature Methods, and have been recognized with several best paper awards at RECOMB, top AI conferences as well as the Google Faculty Award.
Accountable machine learning for safe genome engineering and biotechnology
I will discuss how we can use machine learning (ML) to design safer and more precise biotechnologies, and how we can make ML itself more accountable. In the first part of the talk, I will present our works on using ML to model genome editing and to improve the design of CRISPR targets in human cells. We developed a new method, SPROUT, to accurately predict the distribution of damages that CRISPR induced DNA edits could cause. This also leads to a new way to represent DNA information. In the second part, I will discuss our recent works on making machine learning (and deep learning) more accessible and accountable especially for biomedical applications.Takuji Yamada
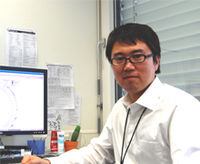
Takuji Yamada is an associate professor at the School of Life Science and Technology, received his doctorate (Doctor of Science) in 2007 from Kyoto University. That year, he was appointed Assistant Professor at the Institute for Chemical Research, Kyoto University. He was then a postdoctoral fellow (2008-2010) and senior technical officer (2010-2012) at the European Molecular Biology Laboratory (EMBL). He joined Tokyo Institute of Technology in 2012 as Associate Professor in the Department of Biological Information. Since 2015, he has served concurrently as Vice President CTO for Metabologenomics, Inc., and since 2016, he has been Associate Professor in the Department of Life Science and Technology, School of Life Science and Technology, Tokyo Institute of Technology. His research interests include bioinformatics, human gut microbiome, and data visualization.
Metagenomic and metabolomic analyses reveal dynamic shifts in gut microbiota along the adenoma-carcinoma sequence in colorectal cancer
Background: Colorectal cancer (CRC) worldwide affects over a quarter of a million people each year. Most sporadic CRCs develop through formation of polypoid adenomas and are preceded by intramucosal carcinoma (Stage 0), which can progress into malignant forms. This process is known to as the adenoma-carcinoma sequence. Detection of early cancers and their endoscopic removal are priorities for cancer control. Accumulating evidence suggests that the human gut microbiome is linked to CRC development. Its comprehensive characterization is of a great importance to assess its potential as a diagnostic marker in the very early stages of CRC.
Study Design: We performed whole shotgun metagenomic sequencing and capillary electrophoresis time-of-flight mass spectrometry (CE-TOFMS)-based metabolomic studies on fecal samples collected from a large cohort of 616 participants undergoing colonoscopy to assess taxonomic and functional characteristics of gut microbiota and metabolites.
Results: Microbiome and metabolome shifts were apparent in cases of multiple polypoid adenomas (MP) and Stage 0, in addition to more advanced lesions (Stage I/II and Stage III/IV). We found two distinct patterns of microbiome elevations. First pattern was represented by Fusobacterium nucleatum spp. whose relative abundances were significantly (P<0.005) elevated continuously from S0 to more advanced stages. Second pattern appeared in Atopobium parvulum and Actinomyces odontolyticus, which co-occurred in Stage 0, were significantly increased only in MP and/or in Stage 0. Metabolome analyses showed that branched-chain amino acids and phenylalanine were significantly increased in Stage 0 and bile acids including deoxycholate were significantly elevated in MP and/or Stage 0. Metagenomic functional analyses showed amino acid metabolism and sulfide producing pathways were found to be associated with CRCs. Particularly, cyclohexadienyl dehydratase gene pheC was highly elevated in Stage 0 (P=0.0000194, q=0.0297). This gene was also identified as one of the top-scored metagenomic markers to distinguish Stage 0 cases from healthy controls.
Conclusion: Our large cohort multi-omics data indicate that microbial and metabolomic shifts occur from the very early stages of CRC development, with possible etiological and diagnostic significance.
See-Kiong Ng
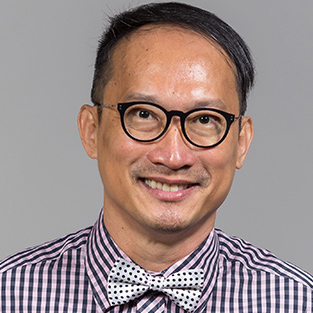
See-Kiong Ng is a professor of Practice at the Department of Computer Science of the School of Computing at National University of Singapore (NUS), and the Deputy Director of the university's Institute of Data Science. See-Kiong's research mission is to develop intelligent techniques to obtain better insights and understanding of the world through computation of data, and to create real-world impact through translating the research outcomes into real-life applications by collaborating with partners from the industry and public agencies. See-Kiong started his research career as a bioinformatician in the mid-1990s. He has since been applying what he had learned from bioinformatics to a wide array of other application domains, with more than 100 papers in peer-reviewed journals and conferences.
Time Series Anomaly Detection using Deep LearningTime series are a fundamental data type for understanding dynamics in real-world systems and their underlying processes. While fine-grained temporal measurements of biological systems may be relatively expensive to obtain to-date, we can learn from other real-world systems to investigate how time series may be a key for unravelling the complexities of systems biology. For example, in the medical domain, time series data are becoming common with the advent of medical devices for physiological monitoring. In many real-world physical systems such as smart buildings, factories, power plants and data centres, the prevalence of networked sensors and actuators has also generated substantial amounts of multivariate time series data for these systems. Detecting anomalous patterns in such time series is a challenging problem due to the complication of the temporal dynamics of these complex systems. We present our ongoing efforts in using deep learning for detecting "irregular irregularities" in univariate medical time series data, and anomalous events in multivariate IoT (Internet-of-Things) sensor data. While anomaly detection is a relatively less-studied topic in bioinformatics, detecting anomalous patterns in time series can potentially lead to useful insights and may find wider use in systems biology as fine-grained time-series biological data become available in the future.